Data driven analytics identify drilling sites close to existing infrastructure
Essentially every company operating in the oil and gas industry is aware that digital transformation and digitalization are a high priority at all levels, from boardrooms to rig platforms. In my role, I work directly in support of industry professionals using digital technologies to improve efficiency and efficacy in upstream environments, most notably within exploration and production (E&P). In our data-driven world, the rate of change is accelerating, as companies, individuals and teams see that machine learning and digitalization can replace time-consuming and monotonous tasks with data-driven systems that enable both cost reduction and new opportunities to add value.
Digital transformation, particularly within a geoscience context, has the power to make less biased volume estimates and more data-driven decisions in E&P. While there’s a real and tangible buy-in relating to the concept of digital transformation in general, most operators now understand adoption is an integral part of their future success. However, when viewed from the perspective of the technology being deployed, its full adoption is still comparatively low. This article will outline the reasons for this, but as a software vendor, we are rapidly working to implement a meaningful change.
Artificial intelligence is changing the energy sector. The digital advancements witnessed by those operating within the sector over the last five years have been nothing short of phenomenal. From beneath the surface, decades of data have been collected, and this knowledge is now at the fingertips of domain experts and decision-makers. The data are being paired with artificial intelligence (AI), cloud computing and modern data analytics technology. Today, operators can undertake geoscience studies to support E&P activity at a pace not previously possible. The next generation of geoscience tools has made significant strides, combining first-hand domain experience with the monumental advancements in data science.
Operators now have a much clearer picture available on critical geological factors. These include factors such as reservoir architecture, rock and fluid property distribution, which have vast potential to reduce exploration uncertainty using insights from AI assisted methods. This innovative technological insight is now available for companies to use and exploit, and it’s a matter of when, not if, they do so. During the last several years, alongside other service providers, Earth Science Analytics has made significant progress in providing operators the tools to use machine learning for their geoscience tasks. From petrophysics to seismic inversion and seismic structural interpretation, digital transformation within geoscience is advancing what can be done at an extremely high level and pace.
The biggest disruption has been the technological progress in the field of AI. Simply put, AI is changing the manner in which the upstream energy sector operates. It is directly altering the landscape of geoscience, leading to numerous benefits for those involved with E&P activities. Not only is it changing what can be seen and done, it is changing how geoscientists interact with data. The potential is there for organizations to make more informed decisions that are faster, better and more accurate than ever before. AI technology can be used to reduce the number of non-commercial well bores and to increase the recovery from producing fields. Once these automated AI-assisted workflows are in place across the industry, it will lead to reduced first oil delivery times and enable projects on a larger scale than ever before. This is, by nature, paired with reduced human bias, leads to better-informed decisions and increased E&P success.
EARTHNET
Traditional seismic inversion methods are time-consuming, resource-intensive and limited by the requirement for highly trained practitioners. Earth Science Analytics understood the challenge and developed EarthNET, an AI-based software for geoscientists that uses deep learning to help geoscientists overcome the time and learning-curve barriers.
The introduction of EarthNET software provides a solution that delivers faster, cheaper and more accurate predictions, using AI to identify rock and fluid properties in the search and recovery of oil and gas resources, Fig. 1. Machine learning combines new methods with algorithms that gain intelligence from all available data, supporting and enhancing data-driven decisions. Ultimately, this helps to increase the efficiency and accuracy of quantitative seismic interpretation activity of those operating in the oil and gas industry, as the real, true value of data can now be fully extracted.
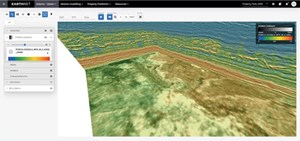
Those using the software can easily leverage advanced no-code AI and data-science technology to maximize the value of their data, while also building strong foundations to support any future decisions.
The software helps to connect energy company users with their internal and external data assets via high-performance computing resources and AI-powered geoscience software applications. This connectivity and the integrated applications allow EarthNET users to break out of the data and discipline silos and embrace truly integrated, cross-disciplinary data analytics workflows. The integrated system allows users to seamlessly access and use all the relevant data to gain new insights, produce 3D property models, and compute data-driven probabilistic volumes for prospects and fields.
Geoscientists now have the tools available to predict reservoir properties directly from seismic data. The models are trained on a combination of well and seismic data, augmented with synthetic seismic responses of nearby or analogue wells, and are used for direct prediction of rock and fluid properties in 3D, including quantification of prediction uncertainty. Access to wide-ranging contextualized data from multiple sources, all in one place, is the key to ensuring that we have the fullest possible picture of what lies beneath, Fig. 2. The days of lacking a first-hand visual perception of rock and fluid-property distribution are over, meaning that operators are no longer approaching their E&P operations blindly or using best-guess estimations to drive decisions.
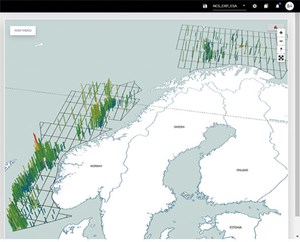
Automatic seismic interpretation. The interpretation of faults, horizons and geobodies from seismic data has always been intriguing, yet time-consuming and complex tasks for practitioners. Its success, or failure, has the potential to impact license rounds and exploration target evaluations, reservoir analysis, and potential drilling hazards and regional studies. Because of this, its importance should never be overlooked. Depending on the size of the survey, previous manual seismic interpretation would have taken weeks at best, or sometimes even months at worst. However, over the last few years, we have worked hard to deliver what we refer to as automatic seismic interpretation. With automatic seismic interpretation now available to operators, the tools are there for faster, higher-quality, data-driven seismic interpretation, providing turnaround time of a few hours, with a cost-effective intuitive software platform that also quantifies interpretation uncertainty, Fig. 3. This digital transformation technology directly uplifts the accuracy and efficiency of workflows, supporting future E&P activity as a result.
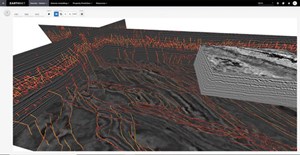
Barriers to entry. While ongoing digital transformation continues to take place at pace, barriers to entry remain, most notably in relation to geoscience’s software requirements. In terms of sector challenges hindering full adoption, there remain several hurdles to overcome. The most glaring is perhaps what is an ongoing transitional phase in terms of legacy software and the need for this to be transitioned to cloud-native solutions. This in turn leads to several connectivity challenges, but one which those in the industry are attempting to address.
Most E&P operators are using legacy software designed for desktop deployment. By their very design, these have traditionally been built with their own data storage systems in place. They are not open for third parties to access and manipulate the data, meaning that the information remains controlled by the original software company. To get around this challenge, any future digital solution must be based upon using data platforms that are fully open, and paired with cloud-native solutions.
The introduction of the OSDU Data Platform will go a long way in supporting this requirement, not only for E&P operators, but for software vendors as well. An open data platform will enable any software provider to deploy its products with full connectivity to the platform and to all the data, via application programming interfaces. Its full adoption will be a significant step-change for the energy sector.
However, this change will take time, as operators large and small will have to completely overhaul how they have previously undertaken IT activities. However, we have first-hand knowledge that several operators are working hard to implement this as fast as they can, a move that can only be viewed as a positive. The digital opportunities available are plentiful. Once operators have systems in place to take full advantage, this could lead to increased success for future E&P activity.
Identifying missed pay zones. One of the most notable aspects of recent digital transformation, particularly in relation to geosciences, centers around the so-called missed pay prediction. Missed pay opportunities can be attributed to several factors, including insufficient data quality and coverage, poor logging runs, lack of evaluation when the initial discovery was made, or a reduction in the knowledge available following company mergers or takeovers. By identifying missed hydrocarbon pay zones among drilled wells in mature basins, operators can be given a second chance to find additional oil or gas accumulations, in a streamlined and efficient way.
Most of the easy-to-access hydrocarbons within existing fields are already being produced, and a significant amount of aging infrastructure is nearing the end of its operational life. Therefore, it’s urgent to find new barrels that can postpone decommissioning. We published our own missed pay findings, based upon our own machine learning technology. The workflow activity was performed within the EarthNET software, using AI and cloud-native technology. With EarthNET’s well-data analytics module, geoscientists can now rapidly predict high-quality rock and fluid property curves and predict missing logs within wells. Geoscientists can apply machine-learning models to predict reservoir properties directly from wireline logs, core data, fluid data and CPI’s. Machine-learning methods for property prediction in wells will support geoscientists to carry out projects efficiently, and at large scales.
By identifying missed pay within exploration wells or assets that are already producing oil or gas, the potential is there to increase reserves, locate and produce cost-effective hydrocarbons, and even deliver new exploration targets to operators. An example of the missed hydrocarbon pay project is available within the accompanying case study.
Looking to the future. Earth Science Analytics’ mission is to provide high-quality data-driven petroleum-geoscience solutions to those operating within the E&P industry. The next-generation E&P software is cloud-native and also deployable on premise or as hybrid solutions. These solutions are real collaborative environments that put data and insights at the fingertips of domain experts and decision makers. These storage and computing resources are available on demand, enabling organizations to rapidly scale up or down, relative to business needs.
We strongly believe that our solutions enable petroleum geoscience teams to work more efficiently, with greater precision and at lower cost, compared to what could have previously been achieved through traditional methods. Our solution ensures that more data-driven decisions can be made, ultimately leading to more profitable decisions. It’s an exciting time to be operating in the sector, as companies become increasingly aware of the availability and potential of new enabling technologies.
The ongoing digital transformation taking place in the energy sector is advancing at an accelerated pace. New opportunities are being identified. However, while barriers to full entry remain, companies that recognize the potential and move rapidly in their adoption of the latest technology will reap the greatest benefits. By fully embracing machine learning, AI and data-driven decisions, operators have the potential to positively impact their company’s profitability. As a service provider, we are excited about what the coming years hold, as digital transformation continues to take root and flourish. It’s a future that we are immensely proud to be a part of and look forward to continuing our role in driving and facilitating ongoing digitalization to help shape the energy sector of the future.
CASE STUDY
Earth Science Analytics were selected by the Norwegian Petroleum Directorate and the British Oil & Gas Authority and Oil and Gas Technology Centre, to deliver the first ever cross-border machine learning project on missed pay. The objective was to predict oil or gas missed pay intervals amongst thousands of wells on a basin scale, leveraging cloud-native big data analytics and machine learning technologies. Operators from the UK and Norway donated over 30,000 km of wireline data that Earth Science Analytics used to predict missing CPI curves and identify over 400 exploration opportunities.
An exploration workflow catalyst. A typical exploration team can deal with 20% of their available data before they must make a drill or drop decision on a prospect. At Earth Science Analytics, we leverage all the available data and enhance them with analogues and or synthetic data, to reveal new insights and uncover viable drilling prospects that may have been overlooked. A collaborative cloud environment brings new insights to old concepts, captures expertise and allows data to be explored with more agility. Thanks to the “on demand” access to computers via the cloud, our work meant we were able to generate rock- and fluid-property curves, bad-hole and QC flags in moments. Using semi-automated tools to edit wells at both single-well and multi-well scales, the result was a data bank of 70 different types of data, spanning 90 million depth indexes.
Identifying close-in drilling sites. From here, we used our wells tool to predict missing logs and then had proven oil finders review the results to ensure we had realistic predictions. By giving our exploration team the tools and data they required, we provided them with the opportunity to take a second look at the subsurface. Using EarthNET for identifying and ranking pay zones, we found over 400 well candidates, located near existing infrastructure, that we believe are worth a second look. These included misreported wells that were tagged as dry, that have potential to trigger new exploration activity in mature areas and provide much-needed new barrels into existing infrastructure.
- Coiled tubing drilling’s role in the energy transition (March 2024)
- Digital transformation/Late-life optimization: Harnessing data-driven strategies for late-life optimization (March 2024)
- The reserves replacement dilemma: Can intelligent digital technologies fill the supply gap? (March 2024)
- Digital tool kit enhances real-time decision-making to improve drilling efficiency and performance (February 2024)
- E&P outside the U.S. maintains a disciplined pace (February 2024)
- U.S. operators reduce activity as crude prices plunge (February 2024)
- Applying ultra-deep LWD resistivity technology successfully in a SAGD operation (May 2019)
- Adoption of wireless intelligent completions advances (May 2019)
- Majors double down as takeaway crunch eases (April 2019)
- What’s new in well logging and formation evaluation (April 2019)
- Qualification of a 20,000-psi subsea BOP: A collaborative approach (February 2019)
- ConocoPhillips’ Greg Leveille sees rapid trajectory of technical advancement continuing (February 2019)