What's New in Exploration
What do you control? Did you read the story that Smart TV’s were recording what you watch and even reporting it to a demographic agency, which uses statistics, specifically neurologic routines, to predict what you will do next? Where is the line between technology, digital information processing, and your decision tree?
The ultimate explanation. Once, when I was serving as V. P. of E&P technology for a large quasi-independent, we went on a trip down the neurologic “yellow brick road.” Neurologic in this context means letting the computer substitute its brain for yours, based on what you tell the robot your expectations are. I was asked to explain this approach to my chairman of the board, so I gave the following analogy, circa 2002.
“Imagine a car traveling down your home street at 5 p.m. each day. Every day, at 5 p.m., the same car passes in front of your house while you stand at the window. You note this on Monday, and every other day of the week. By Friday, you tell your wife, there will be a car passing here at 5 p.m. She watches and, sure enough, there is a car. She had asked, “what color?” You did not record that, but it was blue on Friday. Therefore, you conclude that, because you have a house, or a window, or it was 5 p.m., or that you were standing there, one of those acts had caused the car to pass in front of your house. Satisfied, you sit down. Your wife asks more irrelevant questions on causation—what was the brand, type, number of doors, and how fast? You did not know those things either. ‘So, anybody could have told me that,’ she says. You go back to your TV room and claim it was the wife that caused the TV to be on at 5:05.” Now you understand neurologic computer routines.
Current usage. However, neurologic programs are an accepted method for seismic exploration mapping on workstations and production analysis of historical reservoirs. We have gone way past three-point problems and auto horizon picking (e.g. Stratimagic). It is understandable to use such tools when a seismic-rendered map, or volume you can fly through, contains millions of data bits. However, more important to the problem at hand is how close to the least amount of information can you go?
That is the whole point of using most computer approaches. The former president of Gulf Oil used to say that “when you have all the answers in exploration, it is because someone else owns the oil,” (James Lee, 1983). However, how far can we stretch this technology, to imply answers not commonly found in seismic or geologic data?
Examine this project with the University of Texas. Here, the investigators were provided a 3D marine data set by Marathon Oil and partners, and a handful of wells (cores, samples, petrophysical logs and production data), and asked to create a neurologic system that could predict dynamic data, i.e. reservoir flow parameters and other engineering suppositions from seismic data.
It was a big challenge, but supported by DOE and the industry, particularly BP and Chevron. The test was to see if a data population of 3-5 [wells] could be extended on the seismic. Since I was the project manager at the time, it was amusing to hear each expert tell me that their area had limitations and error concerns, but not the other’s areas. Example, the seismic was perfect to the engineers and geologists for this study because MOC told them they had reprocessed the 13-year-old data. We all agreed that the computer routine for the neurologic derivation would be the deliverable.
Note this abstract from “Multi-point statistics for modeling geological facies of deepwater reservoir—a case study in the Gulf of Mexico,” authored by Sanjay Srinivasan and Lesli Wood while at the University of Texas at Austin (both authors are now at other universities), and reflected in the summary slide on this page, taken from a RPSEA presentation:
Modeling a deepwater turbidite reservoir is very challenging, due to the presence of complex and heterogeneous geological structures. A lot of effort has been expended to model these typical reservoirs, since they account for a large amount of oil production and reserve. A conceptual model that consists of meandering channels, levees, splays and mudstones is constructed for a real deepwater reservoir in the Gulf of Mexico. A geostatistical modeling workflow is then developed, accounting for the uncertainty in model parameters, such as rock type, permeability and porosity. The workflow mainly has two steps: 1) multiple-point statistical modeling of curvilinear channels in lobe deposits to form a two-facies model; and 2) object-based method to add the other two facies, levees and splays, and to depict erosion of these facies. A suite of models is developed following this workflow, and connectivity analysis of the resultant models is performed.
The Final Report for NETL can be found at http://www.rpsea.org/projects/08121-2701-03/.
I commend efforts to perfect statistical analysis from historical data. They can be good tools, if we do not rely too much on them for judgement or driving cars.
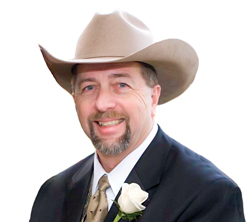
- Applying ultra-deep LWD resistivity technology successfully in a SAGD operation (May 2019)
- Adoption of wireless intelligent completions advances (May 2019)
- Majors double down as takeaway crunch eases (April 2019)
- What’s new in well logging and formation evaluation (April 2019)
- Qualification of a 20,000-psi subsea BOP: A collaborative approach (February 2019)
- ConocoPhillips’ Greg Leveille sees rapid trajectory of technical advancement continuing (February 2019)