Realizing intelligent, autonomous operations in well production
Imagine if every production asset across your wellsites had a dedicated surveillance engineer continuously monitoring and managing its performance. You could keep all your assets performing optimally, and significantly reduce the risk of failures and shutdowns. Such an allocation of resources may seem infeasible in today’s operations, where just a handful of engineers are tasked with watching over and responding to alarms from hundreds of wells.
But now, technologies like artificial intelligence (AI) and machine learning are changing what’s possible in upstream production. These technologies allow you to cost-effectively scale and deploy previously inaccessible intelligence, so no asset is left unattended. This shift to more intelligent and autonomous operations can help you reduce downtime risks. And it can help you dramatically improve your production efficiency.
Overcoming upstream challenges. The nature of upstream oil and gas production has always presented challenges toward implementing widespread automated operations. Wellsites are remote and sprawling, and sometimes difficult to access. Practical constraints, such as cost, can limit the level of instrumentation, control and intervention. Evolving conditions over time also require adaptive methods that can make automated processes simply too costly or difficult to implement using current methods.
However, given the challenges you face as a producer, you may feel you have little choice other than to evolve to more intelligent, automated operations. Not only has the oil and gas industry been among the hardest hit by the pandemic, but such unique technical challenges in the field, require new solutions.
One such example in operations is that engineers are often overwhelmed by too many alarms for too many production assets. This is because the alarms that they monitor operate on tighter bounds, and track whether an asset is operating in an optimal region—hence requiring regular adjustments as things evolve.

Not surprisingly, because they face a flood of alarms, operators or production engineers can, and do, miss important events that lead to asset and production downtime, through equipment damage or unintended trips due to event escalation. They also typically only learn about events after they happen—meaning they're more likely to address problems reactively, instead of proactively. Figure 1 shows a challenging electrical submersible pump (ESP) well with multiple gas interference events. Within the course of 7 months, there was cumulative downtime of about 100 days, almost 100 stop-start cycles (Hz = 0), and a total of 4 days of the system being in stressful low flow conditions.
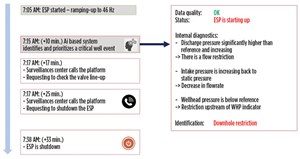
Consider how the tracking and prioritizing of events occurs in most operations. This activity is still largely manual, from detection of what is going on, to closing the loop on actions. Figure 2 shows a timeline of an actual incident on a high-value ESP well, where a real-time AI-based detection engine was tested. The engine was fed real-time signals from the ESP system, such as pump discharge and intake pressures, motor speed, current and temperature, and wellhead pressure. It also was engineered for robustness, with the ability to accommodate different combinations of available measurements, and account for data quality issues, such as missing, frozen and faulty sensor data.
In this incident, the system was able to raise an issue during restart just as early, if not earlier, than best-in-class experts. The solution balances sensitivity that can lead to false alarms and gathering of sufficient evidence before raising the flag. While the solution demonstrated significant value by providing an early alert of an existing critical event, there were still 23 minutes from the point of detection to shutdown, due to the use of largely manual processes. Taking it a step further, could the system be intelligent enough to diagnose the situation, recover on its own, and actually prevent a costly shutdown?
By helping operators resolve critical well events earlier, such solutions also help extend the life of the pump and maximize its production output. During the course of normal operation, ESPs will be subject to multiple stressful events, as well as the normal wear and tear associated with a running mechanical device. The combination of the mechanical stress caused by critical events, such as low flow, and multiple starts/stops, and the normal wear and tear, contributes to the eventual failure of the pump. The longer the duration of the critical events, the larger the stresses on the pump.
This is depicted in Figure 3, on the top timeline, as a red operating zone. The quicker you are able to detect the event and resolve it, avoiding the red zone, the less stress the pump will experience. And that increases the probability of running the pump longer. Over the life of the pump, this results in less intervention costs and increased production time.
Meanwhile, the oil and gas industry also is contending with the challenge of mounting retirements. And as skilled, seasoned employees leave the workforce, they’re taking with them decades of critical knowledge about production assets and processes. Deploying more intelligent production capabilities—where appropriate within your ecosystem—can help you overcome these challenges by capturing crucial process knowledge and enabling higher levels of automation within the control system at the edge.
Intelligent automation at the edge. Intelligence can come from multiple sources. The industry has a rich history of modeling and simulation tools, and operations know-how. More and more today, every solution has to seek the optimal balance between this domain knowledge and machine learning. Historically one or more of these elements are disconnected; what determines the success of more decentralized intelligence, is effectively packaging, deploying and maintaining these elements at scale.
These solutions can slide right into a production asset’s IoT-enabled control panel rack and remote terminal unit (RTU), and be managed centrally from the cloud with over-the-air updates. By deploying this intelligence at the edge, you can get the required response times that are needed for closed-loop automation and optimization. Advanced automation can be done in a reliable manner, without being susceptible to factors like wireless communications disruptions, bandwidth limitations and cost.
Back to the previous ESP example—how can a system not only identify events like the best-in-class experts, but also resolve the events more quickly, reliably and optimally? Like a self-driving car that avoids collisions by detecting risks and performing corrective maneuvers, such AI-based solutions deployed in the control system can recognize high-risk situations by constantly evaluating the probability and severity of issues like low-flow events, and take action immediately in real time. Because ESPs are located downhole, they require adequate flow for cooling the motor and pump. In a low flow situation, a significant amount of energy can potentially be released locally around the ESP, requiring immediate attention to resolve. The solution can act by adjusting equipment operations such as ESP speed or other valve manipulations, based on the specific type of low-flow event that it detects, constantly monitoring the impact of the adjustments based on multiple criteria.
This kind of intelligent decision-making mimics a “super operator,” who can prevent situations from escalating to a point where they cause equipment to fail or trip protection limits, and result in costly downtime. Also, because the system can proactively make control adjustments in the early moments before conditions worsen, it can protect production assets and extend their useful operating life.
Today, with limited resources, operators are having to prioritize which wells they need to pay attention to, based on metrics like production rates and workover costs, while leaving the lower-tier wells to trip and lead to prolonged shutdowns. However, in an era where every bit of efficiency needs to be harnessed, such AI-based solutions that scale could help operators avoid having to make these drastic trade-offs. Finally, the solution’s performance is benchmarked continuously and evaluated by experts, which is critical to building trust between the user and the system.
Higher level of knowledge. When operationalized at scale, these solutions can also improve the management of production assets by providing a higher level of decision support to operations experts. At a centralized level, this allows you to immediately begin capturing, prioritizing, resolving and classifying events. By encouraging this culture in well-designed workflows, a treasure trove of knowledge can be accumulated over time and be used to continuously improve. In the ESP case, as more events are validated and properly catalogued, supervised learning techniques can be used to develop or retrain engines to improve performance metrics.
Also, captured knowledge can then be used across your enterprise, at a level of granularity that matters. Now, instead of losing valuable “tribal” knowledge as workers retire, you collect, retain and share knowledge across your workforce —including potentially newer, less experienced workers—to drive better decisions. This approach also can transform how production personnel do their jobs.
But how? Because the solution, when embedded in the control system, can monitor and respond where appropriate, operators can focus better on mid-to-long-term value-added activities, such as planning of maintenance operations and production optimization strategies. And, as more intelligence is made accessible in the ecosystem, the gap between production engineers and operations will narrow over time. This will enable greater collaboration between the teams and help harvest previously untapped efficiencies.
Additionally, there are elements of the AI-based solution that can learn continuously about each well and its events. With this mechanism, the solution will adapt and eventually be able to improve its decisions to better solve problems, based on each well’s unique history. This allows each well to be controlled as if a dedicated surveillance engineer had been continuously monitoring and supporting it for years.
Reimagining wellsites. Real-time, intelligent solutions at the cloud and edge are already being tested, helping drive better decision-making. They are designed for maximum impact and minimal disruption, with the ability to scale to many assets with minimal set-up and maintenance over time. And ongoing field trials continue to yield positive results, with AI-based solutions being used in the cloud to detect and prioritize events, and at the edge to autonomously resolving critical events and self-improving improving over time.
Soon, intelligent solutions like these will be a competitive necessity for producers that want to not only improve their performance and profitability, but also retain critical operations knowledge before it walks out the door.
- Advancing offshore decarbonization through electrification of FPSOs (March 2024)
- Digital transformation/Late-life optimization: Harnessing data-driven strategies for late-life optimization (March 2024)
- The reserves replacement dilemma: Can intelligent digital technologies fill the supply gap? (March 2024)
- What's new in production (February 2024)
- Digital tool kit enhances real-time decision-making to improve drilling efficiency and performance (February 2024)
- U.S. operators reduce activity as crude prices plunge (February 2024)